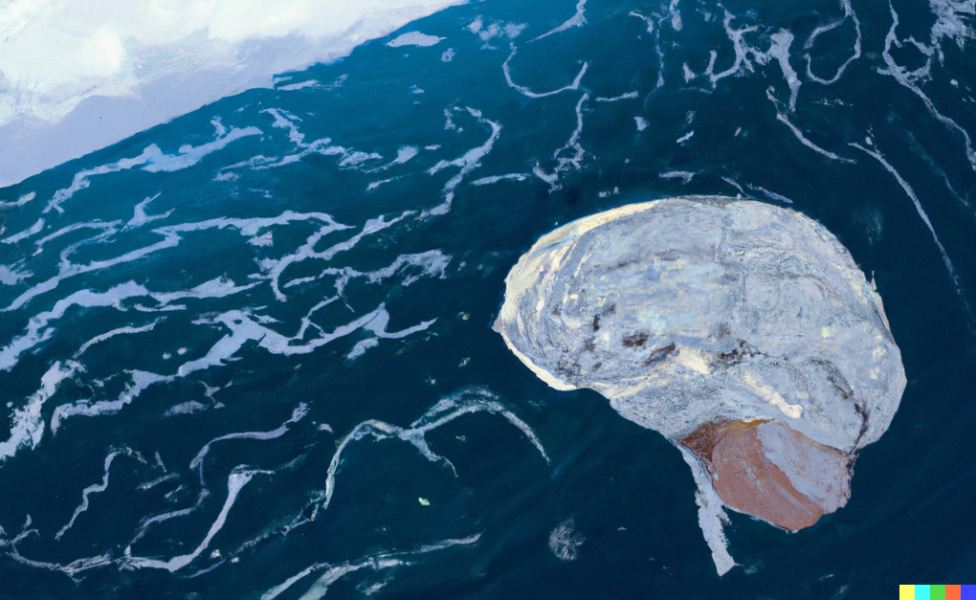
AI Atlas #24:
Liquid Neural Networks
Rudina Seseri
🗺️ What are Liquid Neural Networks (LNNs)?
Liquid Neural Networks (LNNs) represent a type of Recurrent Neural Network (RNN) that operates sequentially, organizing time-series data to retain memory of past inputs and dynamically adjust behavior based on new inputs.
The term “liquid” suggests a dynamic and flexible neural network architecture. Just like a liquid can take the shape of its container, a Liquid Neural Network can adapt its structure based on the data it encounters and the tasks it performs. In doing so, LNNs aim to obtain deeper insights from a smaller, simpler set of connections.
This adaptive architecture enables LNNs to learn on the job, setting them apart from traditional neural networks that rely solely on pre-trained data. LNNs distill tasks and drop irrelevant information. Their fluid nature and real-time adaptability make LNNs exceptionally well-suited for tasks involving continuous sequential data, offering improved interpretability (the ability to understand how they work) and more efficient processing by utilizing fewer, richer neurons than in a traditional RNN.
Although initial research dates back several years, the architecture has entered public consciousness only months ago. In January of this year, researchers from MIT announced that they had applied a new type of machine learning architecture to pilot a drone with only 20,000 parameters. This is a relatively tiny architecture in the world of neural networks, where state-of-the-art models can be millions to billions of times larger depending on use case. However, despite the smaller size, drones equipped with this system were able to effectively navigate complex environments and adapt to new ones with higher precision than existing systems, even with the addition of noise and other obstacles.
🤔 Why LNNs matter and their shortcomings
LNNs represent a significant breakthrough in ML and AI, more broadly, as they fundamentally challenge the current philosophy of “more is always better” in terms of model size and data consumption. They hold considerable significance due to their ability to address limitations inherent to conventional neural networks; for example, unlike traditional networks that process data non-sequentially and require extensive labeled training data, LNNs can adapt and continue to learn from changing data even after the initial training phase. This feature was inspired by C. elegans, a small worm that exhibits complex behavior despite having only a handful of neurons. LNNs thus eliminate the need for initially vast amounts of labeled data and ensure continual adaptation.
Reduced resource intensiveness: LNNs enable the solution of complicated problems with reduced computing power, capable of running even on common industrial microcontrollers such as the Raspberry Pi. Because they do not require as intensive of a training phase as traditional neural networks, they can theoretically also be created with less demanding data requirements, such as learning a language over time via exposure rather than from a large initial training period.
Continual learning & adaptability: LNNs adapt to changing data even after training, mimicking the brain of living organisms more accurately compared to traditional neural networks that stop learning new information after the model training phase. Hence, LNNs don’t require vast amounts of labeled training data to generate accurate results.
Explainability and Interpretability: the “black box” nature of larger, more complicated models is a sticking point of concern as the technology continues to develop. As we discussed with our exploration of MILAN, finding ways to simplify machine learning allows for greater explainability and interpretability. LNNs achieve this with their smaller size and heightened focus on the cause-and-effect nature of specific tasks.
While LNNs offer numerous advantages, they are not without challenges.
LLNs require time-series data: because LNNs are designed around chronological progression, they have not yet been applied to use-cases with static data, such as image recognition or classification tasks.
The technology behind LNNs is young and there are still many unknowns: the limited literature and recognition compared to established neural network architectures pose a hurdle in understanding and maximizing the potential of LNNs.
The efficacy of training lessens over time: Recurrent Neural Networks, of which LNNs are a subset, tend to experience reduced benefit from subsequent additions of data as patterns begin to generalize. This is known as the vanishing gradient problem, and it represents a hurdle for the technology’s longer-term efficacy.
🛠️ Applications of LNNs
LNNs have compelling applications in various domains, particularly those involving continuous sequential data.
Time-series data processing benefits from LNNs’ adaptability, as they can effectively handle time-series relationships and changing circumstances. Time-series data can be used to analyze trends and forecast financial, medical, and even meteorological events.
Natural Language Understanding: representing language as a time-series brings forth unexpected benefits; for example, recognizing the evolution of dialects and vocabulary over time can enhance accuracy in sentiment analysis.
Edge Computing and Robotics: as demonstrated by the drone demonstration at MIT, LNNs are compact enough to be run on microcontrollers such as the Raspberry Pi. This means that ML can be quickly and cheaply applied to a variety of use cases involving robotics and industrial processes, including the development of autonomous vehicles, without the need for cloud processing or external hardware.
In summary, Liquid Neural Networks stand as a groundbreaking advancement in AI technology, potentially redefining neural network design with their adaptability, efficiency, and interpretability. Their transformative potential across various domains is unmistakable, promising a future where AI systems can adeptly navigate intricate real-world challenges while offering a clearer understanding of their decision-making processes.