Generative AI Series Part 2:
Application Prioritization Criteria
By Rudina Seseri and Kleida Martiro
Introduction
In this second installment of Glasswing’s generative AI series, we delve into the Glasswing evaluation framework for assessing investment opportunities in the application layer. The cornerstone of the evaluation framework is a set of five criteria that we’ll outline in detail below, but let us first revisit our breakdown of generative AI tech stack.
As noted in our prior installment, the application layer holds the greatest opportunity for founders and venture investors. Companies dedicated to offering clear, quantifiable benefits with AI-generated data and analyses have the potential to overtake established industry leaders and set new standards. Mirroring the evolution witnessed in the cloud software sector, we foresee a vast array of successful generative AI application companies emerging, far outnumbering the prominent entities present in the middle and infrastructure layers.
Application Area Prioritization Criteria
We have developed a proprietary set of criteria for evaluating the attractiveness of investing in various application areas. By application area, we are referring to industry verticals, such as financial services, or functional areas, such as marketing or software development, where generative AI solutions can be applied and where they can have a significant impact. Our prioritization framework goes beyond mere academic exploration, as establishing criteria is vital in translating our tech stack into high-conviction investments and productive strategy discussions with our founders.
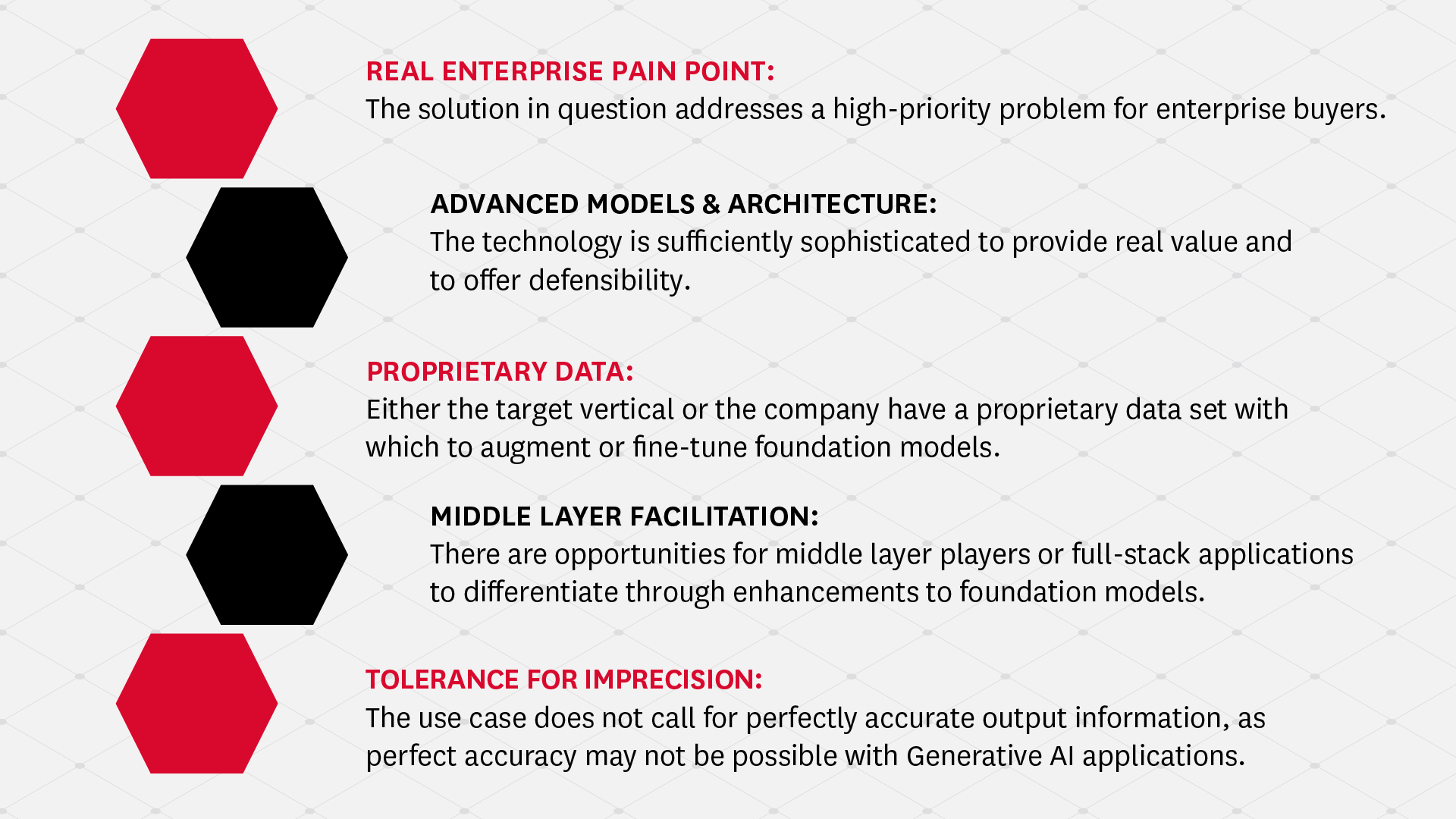
We have established five key criteria for an application area to be deemed attractive for founders building businesses and investors in the generative AI space. While a brief explanation of each criterion is given in the graphic, we are providing our rationale for the inclusion of each below:
Real enterprise pain point – For an application area to be viable, the pain point needs to be very high on an enterprise buyer’s radar, and the budget for addressing said pain point needs to be substantial. A common litmus test for this criterion is to see whether the pain point in question comes up as one of the top two or three priorities for enterprise executives and whether a budget has already been established to address the issue. There are a number of startups coming into the market with “chatbot for X” solutions even though said chatbot will not address one of the top pain points in any vertical or function.
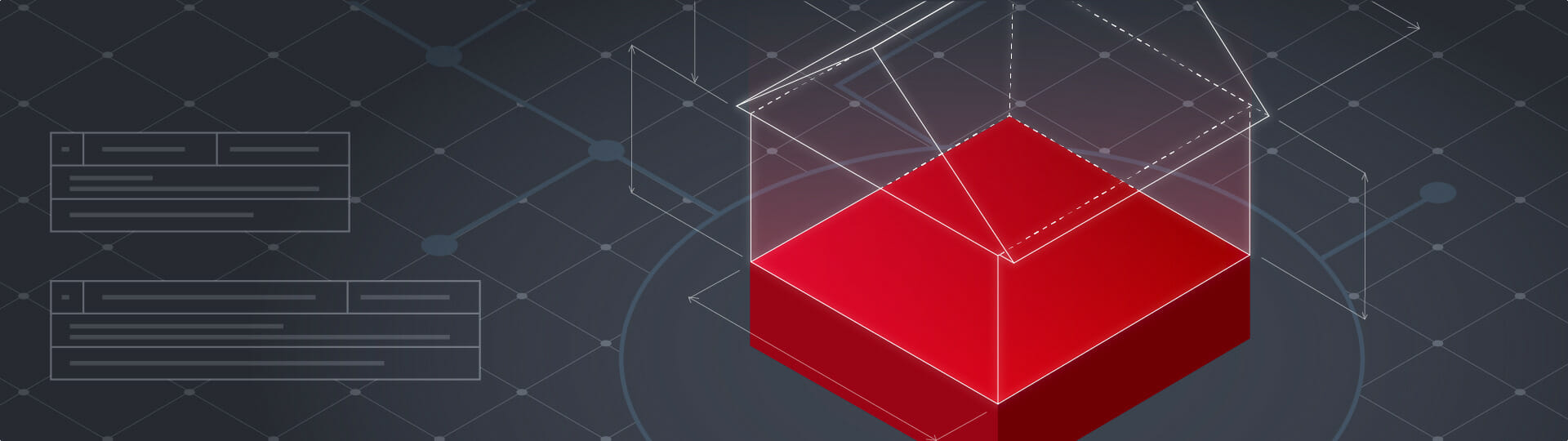
Advanced models & architecture – While it is a powerful tool, ChatGPT cannot fix most (or any) of an enterprise’s biggest problems. In order to provide tangible value in enterprise use cases, emerging application companies need to build or leverage cutting-edge models and deliver the outputs from those models via sophisticated application architecture. Models vary significantly in architectural complexity and parameter count. Understanding the underlying algorithms is critical to finding a model that fits the founders’ specific needs. For instance, for real-time translation tasks, selecting a transformer-based model is appropriate. Some tasks require intricate understanding and complex reasoning, and in these cases, larger models are more effective. Smaller models, while more computationally efficient, can leave gaps in understanding and interpretation. Recognizing these limitations is pivotal to prevent selecting a model ill-equipped for the task at hand. As we shared in our installment on generative AI, we are not investing in shiny front-ends that simply sit on top of ChatGPT, as these companies do not have a viable path to long-term differentiation.
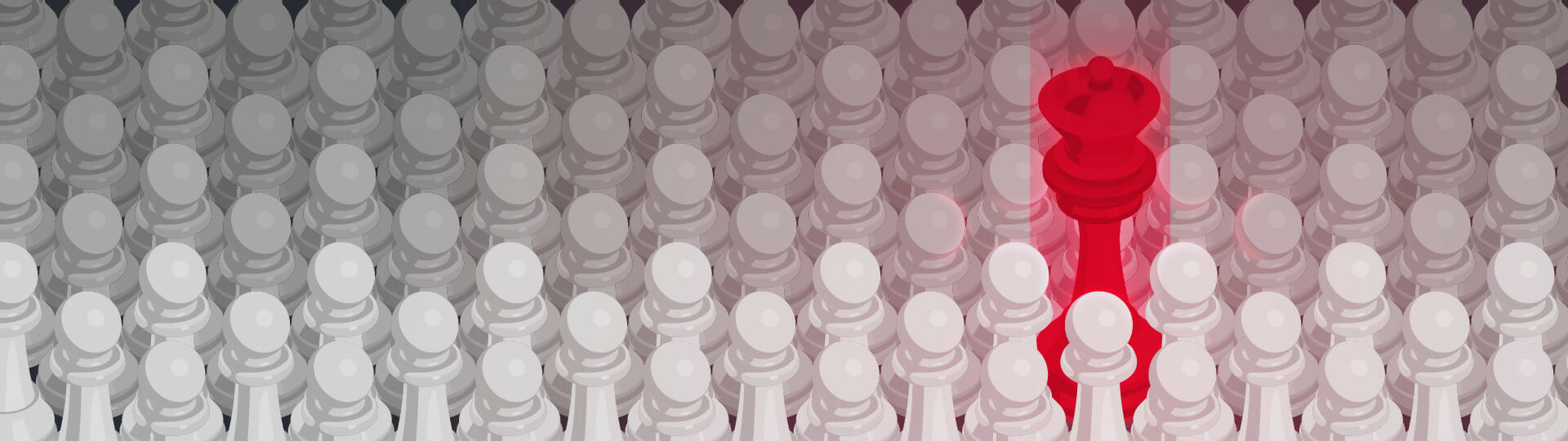
Proprietary data – One of the most compelling pieces of any AI application company is access to proprietary training data, which holds true in generative AI. While there are entire industry- or function-specific LLMs emerging (e.g., BloombergGPT for finance) at the infrastructure layer, our view is that most application companies will differentiate themselves by fine-tuning LLMs with their proprietary data. This proprietary data not only equips them with unparalleled insights and accuracy, but also fortifies them against rapid commoditization. In a world awash with generic data, these unique and guarded data reservoirs will propel an AI application from being merely effective to truly transformative.
Middle layer facilitation – In the above criteria, we hinted at fairly sophisticated tasks, such as architecting a switch between different foundation models based on dynamic end-user contexts or fine-tuning a foundation model with proprietary data. These types of capabilities are precisely those that fall into the middle layer of generative AI and that help application developers harness the power of foundation models in the most effective way for their chosen use case(s). Glasswing predicts that a dedicated group of middle layer companies will emerge over time to provide these capabilities to app developers, but, in the near term, we are looking for application companies to differentiate themselves with some in-house middle layer capabilities. As a reminder, Glasswing refers to companies that have in-house foundation models, middle layer, and front-end (aka application) capabilities as full-stack or vertically integrated generative AI companies.
Tolerance for imprecision – A simple truth about the state of generative AI is that the current technology in the market cannot produce factually accurate outputs in 100% of cases. Accordingly, we are encouraging founders to build in application areas where users can live with some level of imprecision. A large reason why the marketing and sales functions have seen such rapid adoption of generative AI solutions is because those functions inherently operate with high levels of subjective and ambiguous content. Healthcare, on the other hand, requires incredibly high levels of information accuracy for medical and compliance reasons, so it stands to reason that those users will be slower to adopt generative AI solutions and that companies in that space may take longer to scale.
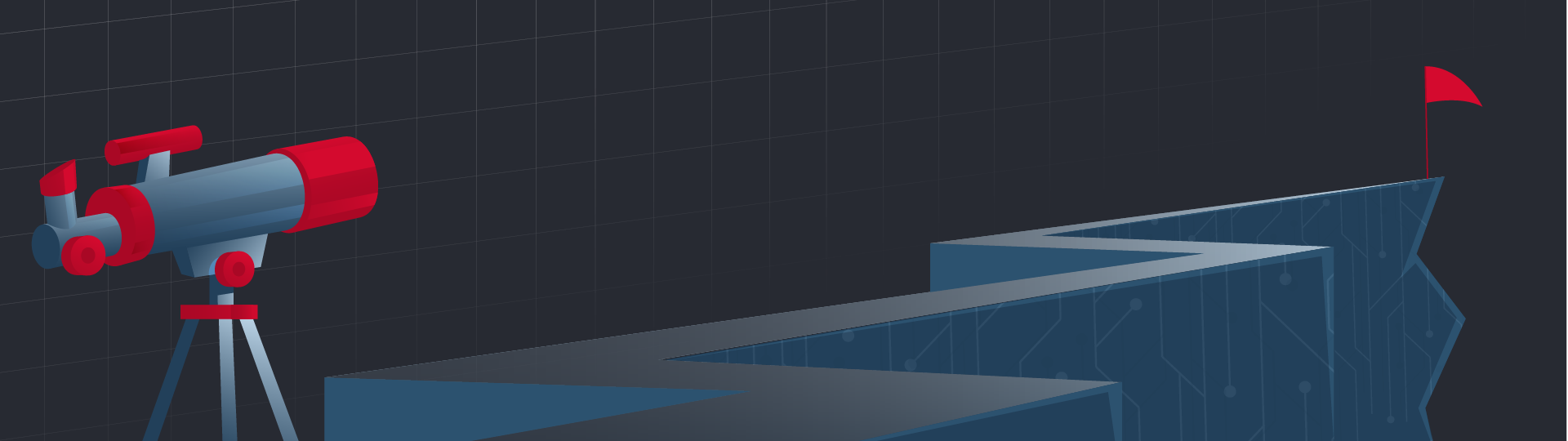
Looking ahead
There are already several application areas that score highly when evaluated against the Glasswing prioritization criteria. As we continue our series on generative AI, we will be diving into a few of the application areas that are of the greatest interest to Glasswing and where we have been making investments over the last few years. In our first deep dive on generative AI in the manufacturing and supply chain sector, you will see our prioritization criteria in action and gain a better understanding for how certain application areas are ripe for disruption.