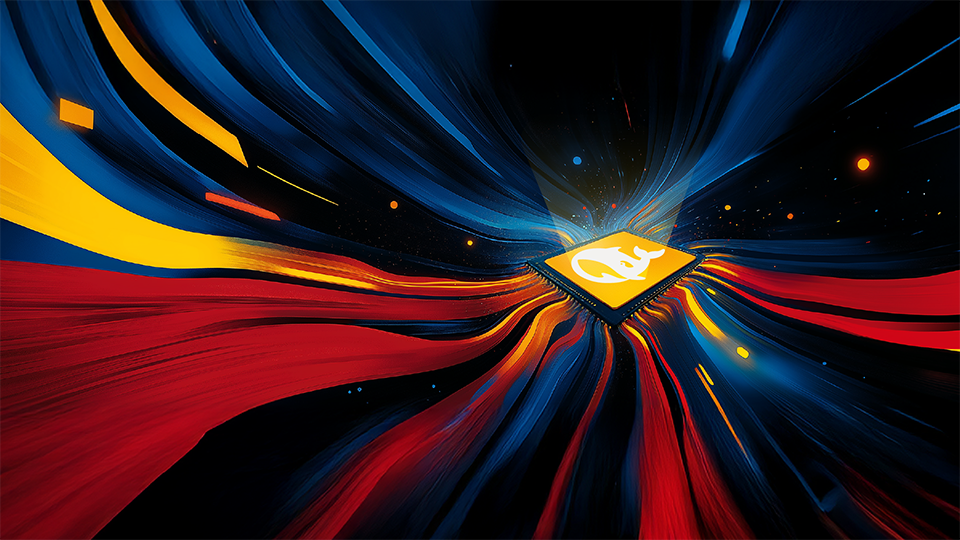
How Glasswing Saw DeepSeek Coming
Glasswing Ventures firmly believes that the most attractive AI investment opportunities exist at the application layer of the AI tech stack. The true power of AI lies not in the generic capabilities of foundation models but in the specific technology designed for unique markets and verticals. When AI-native products leverage training data and are optimized for certain business outcomes, they positively impact industries.
Glasswing has always held the perspective that, in due time, large language models (LLMs) will transition from a premium offering to a standardized utility of AI innovation. Similar to how the foundations of cloud computing became commoditized over time with new innovations and competition arising in the application layer, we will see a similar occurrence with AI foundation models. The release of DeepSeek-R1 underscores both the importance of open-source AI research and the challenge of achieving lasting differentiation at the foundation level.
What is DeepSeek-R1?
DeepSeek-R1 is an open-source LLM developed by DeepSeek, an AI company spun out of the Chinese hedge fund High-Flyer. The model has garnered headlines for achieving state-of-the-art performance across a number of benchmarks while cutting training and inference costs by over 90% when compared to incumbents such as OpenAI’s ChatGPT, Anthropic’s Claude, and Google Gemini. While those following AI have heard about DeepSeek’s prior models, this most recent release cements the company as a formidable player in the global AI race.
Additionally, though the upfront numbers are impressive and maybe even startling, a look under the hood of DeepSeek-R1 provides a clear explanation as to why we are seeing this milestone now and what it means for the future of AI product development.
Out-Innovating vs. Out-Spending
More expensive doesn’t always mean better. Instead, true value lies with technical differentiation. Glasswing invests in early-stage AI-native platforms that bridge the application and middle layers to maintain deep technical defensibility through unique algorithms and data moats. Since the early days, our AI-native companies have recognized the opportunity to disrupt their markets through groundbreaking innovation, driving business value and maximizing ROI for end users.
It is believed that one of the reasons DeepSeek shied away from traditional methods of training LLMs was to overcome one of the biggest obstacles in AI development: resource allocation. Whereas conventional LLMs carry high computational demands, DeepSeek found new ways to train and refine models in a far more efficient manner.
Due to US restrictions on chip buying in China, the DeepSeek team could only access about one-eighth of the standard amount of Nvidia chips required to effectively train LLMs. This scarcity forced creativity, pushing the team to innovate and develop the computation and memory enhancements we list below.
The Power of Smaller, More Specialized AI Models
As generic models consistently fall short in addressing industry-specific or task-specific needs, model specificity has gained popularity in the market. Over the past few years, smaller AI models such as Liquid Neural Networks and Mamba have proven to increase the efficiency and agility of AI models for specific use cases. Models are able to shrink in size without sacrificing capabilities, packing the power of larger systems into more compact frameworks.
DeepSeek embraces this trend through a series of innovative strategies. For instance, while R1 was trained on a number of large corpora, it has been speculated that the model leveraged “distillation,” wherein it calls on the APIs of other LLMs, such as those developed by OpenAI and Meta, and essentially learns from them. This combination of broad training and targeted learning allows DeepSeek to deliver high performance in a more efficient package.
Furthermore, DeepSeek-R1 increases the specificity capabilities of foundation models through its “Mixture of Experts” approach to training. Instead of relying on a single monolithic model, DeepSeek segments its architecture into a collection of smaller, more specialized models, each dedicated to a unique knowledge base. This design allows the AI to selectively activate only the relevant portions of its 671 billion parameters – for example, 37 billion for a given task – based on the input context. The result is a dramatic increase in efficiency, reduced load times, and improved performance.
Open-Source vs. Proprietary
Open-source and proprietary LLMs offer distinct advantages, but both come with trade-offs. Open-source models stand out for their flexibility, transparency, and collaborative potential, all at a lower cost – though they require significant technical expertise. In contrast, proprietary models simplify deployment but often come with less predictable pricing and limited flexibility.
DeepSeek bridges this gap, combining beneficial aspects of both approaches. By creating an affordable yet high-performing foundation model, DeepSeek democratizes AI access for smaller businesses, researchers, and developers. It challenges the exclusivity of proprietary solutions, pushing closed-source providers to lower costs and adopt greater transparency while simultaneously empowering innovation across industries.
Reinforcement Learning Takes Center Stage
Conventional AI models rely on two key training phases: supervised fine-tuning (SFT) and reinforcement learning (RL). SFT uses labeled data, such as step-by-step reasoning processes, where each step is explicitly guided. In contrast, RL focuses on outcome-based learning, where only the final result (like the correct answer to a math problem) is labeled, regardless of the steps taken. DeepSeek places greater emphasis on RL, allowing the model to learn through trial and error. This approach reduces the need for extensively annotated training data, offering a more efficient and scalable solution.
DeepSeek’s innovative RL framework integrates learning into the core of the training process, enabling real-time, context-driven decision-making. By breaking the model into smaller components and iteratively evaluating subsets, DeepSeek ensures precise reasoning rather than mere data processing. This method not only reduces computational costs but also represents a significant advancement in AI training, as the model essentially trains itself with minimal supervision. This self-directed approach marks a major milestone in reinforcement learning, delivering a smarter and faster solution compared to traditional methods.
US vs. China
This topic cannot be discussed without mentioning the ten thousand-pound elephant in the room: China. Given that DeepSeek is a Chinese company, concerns around data privacy and security should be high. If we think about the scrutiny that Huawei and TikTok have received over the past years, DeepSeek should generate loud alarm bells.
Although the model is open-source and the codebase can be closely inspected, a Chinese-based LLM that does not abide by US privacy laws or European regulations like GDPR – not to mention one that is aligned with and supported by the Chinese government – could create major issues for businesses and consumers, leaving their data and privacy compromised, and generate biases not consistent with expected norms. Already, it has been made explicitly clear that all data stored in DeepSeek is being sent back and stored in China, including device identifiers and keylogging data. So, while R1’s can be hosted locally as an open-source model, extreme caution should be used when deploying DeepSeek’s outsourced offerings in sensitive applications.
Security Still Reigns Supreme
After achieving the number-one placement in app stores worldwide on Monday, DeepSeek was hit by a cyberattack, leading the company to temporarily restrict new user registrations. This incident emphasizes that, as with any new technology, security must be made a priority from the start.
Assuming it continues to meet the expected hype, DeepSeek, along with analogous emerging technologies, has tremendous potential to introduce critical vulnerabilities across the enterprise. Attackers may leverage DeepSeek’s AI model as a simple, powerful, and cost-effective tool to better discover and exploit the cyber vulnerabilities of any organization. The open-source model increases the attack surface throughout the AI development lifecycle and model inference, making it easier to generate new threats – such as deepfakes – that can enhance ransomware, theft, and fraud. Its potential negative consequences may even redefine the global norms around data privacy and security.
The Glasswing Perspective
Three years ago, OpenAI flipped the world on its head with the launch of ChatGPT and we witnessed the market shift as more dollars were invested into resource-intensive AI projects. Glasswing remains firm in its conviction that the largest opportunities for early-stage investment lie with AI-native platforms that are able to develop a full-stack application and middle-layer capabilities while maintaining deep technical defensibility through unique algorithms and data moats. We do not view the foundation layer becoming largely dominated by incumbents and open-source disruptors as a negative. Rather, we believe that the development of technology such as DeepSeek will propel our companies forward, encouraging them to innovate faster and stay scrappy while providing them with access to less expensive resources that will allow them to develop faster. The move towards smaller models, introduction of new training methods, general creativity, specificity, and security awareness introduced by DeepSeek-R1 showcase the exciting era of AI development we are in.
At Glasswing Ventures, we remain at the forefront of these latest AI innovations, actively backing visionary, early-stage founders who push the boundaries of possibility to reinvent enterprise and security markets.
Note: This is Glasswing Ventures’ perspective as of the information available on January 28. We will update the post as the story progresses.