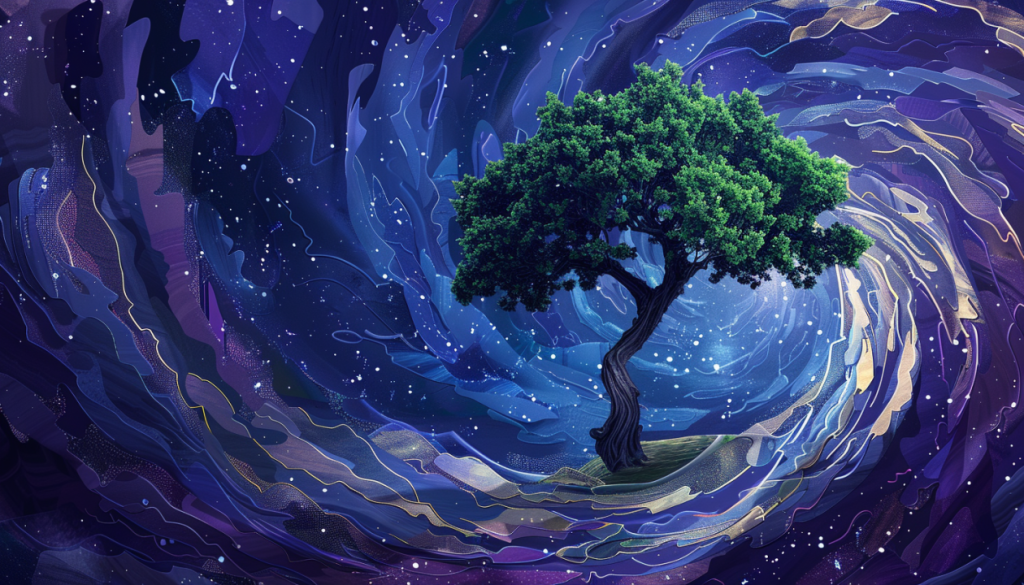
AI Atlas: The Current Landscape of Large Language Models
AI breakthroughs, concepts, and techniques that are tangibly valuable, specific, and actionable. Written by Glasswing Founder and Managing Partner, Rudina Seseri
This past month has been exciting in the realm of Generative AI, with a series of major announcements on new models and capabilities for enterprises, as well as several high-profile talent shifts amid increasing discussion around enterprise safety and security.
AI is a broad term that encompasses a range of architectures and techniques. Within this, Generative AI (aka GenAI), refers to the body of AI techniques based around architectures such as Large Language Models, which are capable of generating human-like text and images. These models consume massive amounts of data and require extensive computational resources at scale, hence the popularity of managed services from providers like OpenAI, Google Gemini, and Anthropic, but many enterprises have chosen to build customized and self-hosted AI systems using open-source models to unlock greater transparency and control.
However, as these foundation models bitterly compete to gain market share in the emerging Generative AI industry, what is it that really sets them apart? What capabilities are table stakes in the current AI landscape, and what is worth tracking for the future? In today’s AI Atlas, I dive into those questions and explore how enterprises should frame their evaluation of LLM offerings.
🗺️ What are foundation models capable of today?
Perhaps the most discussed announcement was OpenAI’s unveiling of its new GPT-4o model, which represents a major step forward for LLM multimodality. I wrote about multimodality in a previous AI Atlas, defining it as the ability of AI systems to accept and deliver content across various forms including text, images, videos, and audio. Unlike some other models that might handle different modalities through separate, specialized systems or modules, GPT-4o integrates all these modalities into one cohesive neural network. This integration allows for more seamless and coherent interactions across different types of media, for example by leveraging product reviews to design new packaging in real-time.
However, ChatGPT is not the only foundation model with new features. Google recently announced their own multimodal capabilities with Gemini 1.5, as did Anthropic’s Claude 3, which includes a niche in AI safety and security. These advancements will continue to push the boundaries of what is possible from Generative AI, while finding new efficiencies to reduce energy and data costs.
Beyond new model capabilities, we have seen a significant expansion of LLMs beyond web-based chatbots and into enterprise-grade formats and applications. For example, Google recently announced Gemini integrations across their product suite, from Gmail to Docs, Sheets, and Meet. Additionally, recent innovations in open-source LLMs such as those from Hugging Face have unlocked new capabilities for democratization and transparency, enabling small, customized models that require less data and hardware to operate effectively.
🤔 What LLM capabilities are most important, and what still needs improvement?
These recent advancements have not only enhanced GenAI as a tool but have also opened up new possibilities for its utilization. With expanded multimodal capabilities and the integration of LLMs into vertical applications, we are moving closer to a future where AI seamlessly integrates into everyday life, empowering both individuals and businesses with unparalleled intelligence and efficiency. While the line continues to blur between individual model providers, long-term value will be driven by model features such as:
- Interoperability with vertical applications: A holistic approach to processing different types of data empowers AI systems to process and respond to more complex and nuanced inputs, making them versatile across domains from content generation to business intelligence.
- Edge computing: As I discussed in a previous AI Atlas, incorporating multimodal AI assistants into edge devices such as smartphones and micro-controllers will revolutionize how AI fits into business workflows, making tasks more seamless and intuitive.
- Resource efficiency: The development of more efficient model architectures saves both energy and hardware costs, lowering the barrier to enterprise-grade AI.
However, GenAI is not a panacea, and it is important to be realistic about their current strengths and weaknesses. The speed of LLM adoption in key business areas, especially those closer to revenue generation, depends on the abilities of model providers to address these shortcomings in a user-friendly manner:
- Hallucinations and errors: Hallucinations, or the tendency of an LLM to confidently output incorrect information, are a persistent issue in AI due to the black box nature of many AI models, data biases, and other emergent behaviors. An understanding of these errors, as well as their downstream impact, is important for AI adoption in more sophisticated use cases.
- Over-generation: GenAI models are susceptible to data quality issues and often have difficulty providing short, quality answers to specific questions without additional prompting. This will result in computational waste and operational complexity, making it necessary for enterprises to develop strategies to filter and manage the generated content effectively.
- Security considerations: LLMs continue to raise security concerns related to malicious use, data privacy, and bias amplification. Mitigating these issues in practices requires coordinated measures such as robust data quality management and the controlled evaluation of outputs.
🛠️ What LLMs are being used for today
Ultimately, designing an effective AI system means selecting specific techniques and models with a clear understanding of the desired end use case. LLMs have already found utility in a variety of business areas due to their strengths in natural language processing and ability to ingest massive amounts of unstructured data:
- Conversational interfaces: GenAI enables humans to engage directly with software without prior coding knowledge, making it extremely useful for building natural language UI.
- Large-scale data summarization: LLMs efficiently distill extensive datasets into concise summaries, enabling quicker insights and decision-making processes.
- Automated content generation: Companies such as Common Sense Machines have built their own foundation models capable of creating rich 3D models from 2D images and plain text, unlocking entirely new workflows for what was historically a highly labor-intensive process.
Stay up-to-date on the latest AI news by subscribing to Rudina’s AI Atlas.