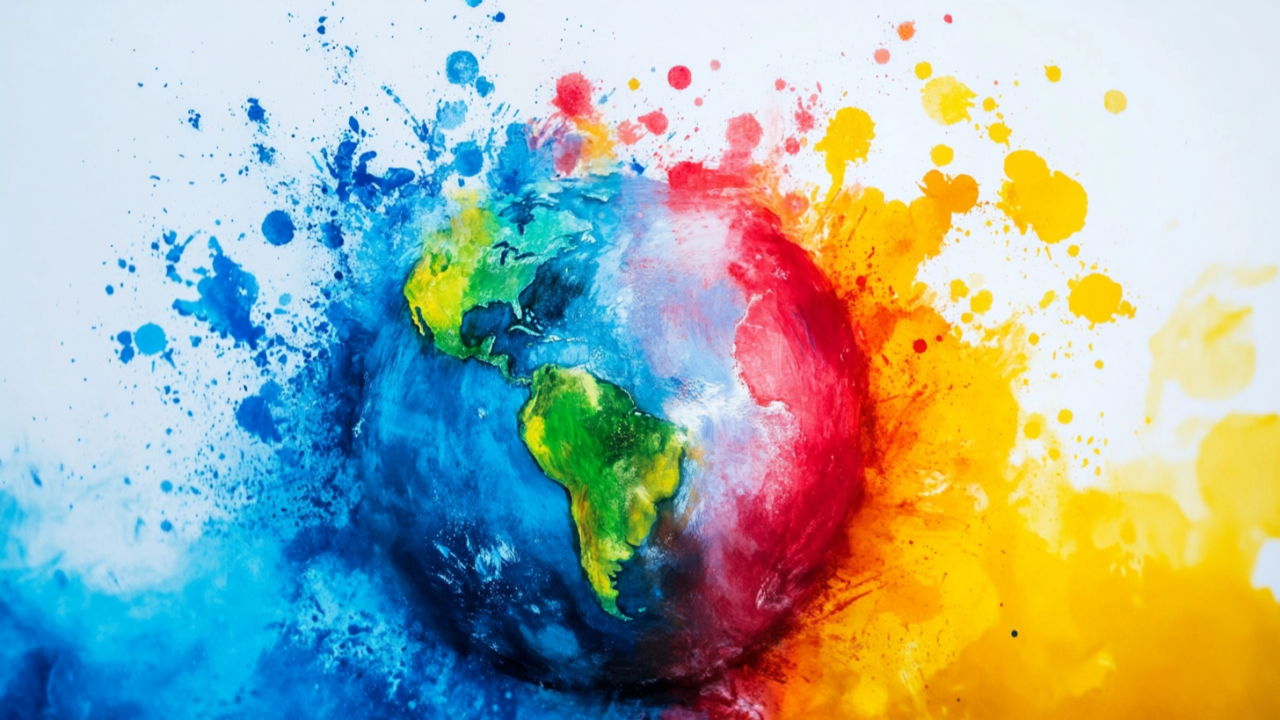
AI Atlas:
How World Models Visualize Reality
Rudina Seseri
Some time ago, I wrote a post outlining a few critical things your children can do that AI could not with regard to logical decision-making. In the year and a half since, AI products on the market have achieved remarkable new benchmarks, from ChatGPT-4o’s groundbreaking image generation feature to ensemble AI agents capable of detecting patterns across disparate datasets. However, there are still areas where even the most performant AI models cannot match human intuition.
Some examples of essential cognitive abilities that modern AI platforms lack include abstract reasoning (seeing the bigger picture), causal understanding (if A then B), and contextual awareness (B happening affects C too). These limitations are rooted in how AI learns; today’s models rely on statistical correlations, allowing them to predict outcomes but not empowering them to truly understand why those outcomes occur. In contrast, humans develop intuitive models of the world through experience and interaction starting from infancy.
That gap, however, is beginning to narrow. This month, NVIDIA announced its new “Cosmos” foundation models, introducing customizable AI reasoning in cyber-physical applications such as robotics and autonomous systems. This is an exciting advancement in a burgeoning field of AI known as world models, which build internal representations of an environment, unlocking far more sophisticated reasoning and adaptability in dynamic real-world settings.
🗺️ What are World Models?
A world model is an AI architecture that builds an internal representation of its surroundings in order to predict outcomes and guide decision-making. Instead of passively recognizing patterns in data, an AI tool equipped with a world model actively simulates context, making it better at reasoning and adaptation.
Longtime readers of this Atlas may notice that this sounds familiar to Physics-Informed Neural Networks (PINNs), where known physics equations are integrated into a neural network’s learning process, dramatically boosting the AI’s ability to produce accurate results. In fact, PINNs are indeed a type of world model — for example, a neural network integrated with the known physical equations of gravity would be useful for developing highly optimized simulations of object movement. In essence, world models develop structured knowledge through experiential learning, ultimately enabling them to infer cause and effect with high accuracy.
🤔 What is the significance of world models, and what are their limitations?
World Models represent a new paradigm in AI reasoning. By building dynamic internal simulations of the real world, these models enable longer-term planning and unlock unprecedented levels of optimization. Traditional AI techniques are constrained by historical data, but world models show promise in being significantly more efficient, scalable, and impactful at providing seamless integration between digital and physical systems.
- Adaptability: A world model builds an internal representation of its surroundings and then learns from that space through direct interaction. This helps the system generalize beyond its training data and adapt to novel situations more efficiently.
- Informed decision-making: By leveraging cause-and-effect relationships as a base, world models can make more informed decisions rather than merely predicting based on statistical associations.
- Higher data efficiency: In theory, world models can learn more efficiently from fewer examples, much like how humans infer general rules from limited observations.
However, while world models are promising, there are a few notable areas for continued research before enterprises can develop conviction behind production deployments in impactful use cases:
- Overspecialization: Unlike humans, who are naturally good at generalized intuition, world models can become overly specialized, making them unpredictable in unfamiliar scenarios.
- Complexity: Simulating entire environments and maintaining these internal representations require significant computational resources at scale.
- Transparency: Some researchers have indicated that further work is needed to improve the interpretability of World Models and make their reasoning process auditable. This would be especially important for applications in regulated industries close to the business core, such as in process engineering automation.
🛠️ Use cases of World Models
Ongoing advancements in causal inference, PINNs, and human-in-the-loop AI workflows are making world models an increasingly promising technology for business applications such as:
- Manufacturing: For example, Glasswing’s portfolio company Basetwo leverages PINNs, a type of world model, behind its no-code AI platform for engineers to simulate and optimize process manufacturing.
- Finance: World models could lay the groundwork for AI-driven investment platforms capable of predicting market movements, modeling economic scenarios, and advanced risk assessment.
- Supply chain: Businesses could use AI-driven world models to optimize supply chains and improve resource planning.
Stay up-to-date on the latest AI news by subscribing to Rudina’s AI Atlas.