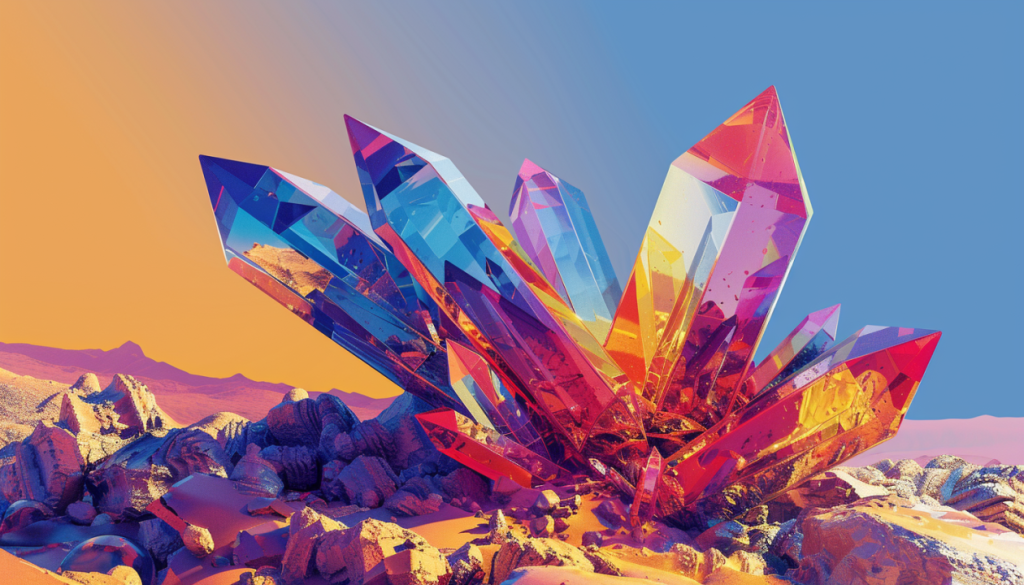
AI Atlas: From Noise to Clarity: Finding New Use Cases for Diffusion Models
AI breakthroughs, concepts, and techniques that are tangibly valuable, specific, and actionable. Written by Glasswing Founder and Managing Partner, Rudina Seseri
One of the most prominent AI innovations in recent years has been the ability to generate lifelike visuals from simple textual descriptions, such as with Midjourney (images), OpenAI’s Sora (videos), or Common Sense Machine’s Cube, which can even generate full 3D models. These AI systems all make use of an architecture known as a diffusion model, which has risen in popularity for various computer vision tasks due to its power in creating high-fidelity outputs, such as detailed images or intricate 3D textures.
However, Diffusion Models are not limited to the visual world. New research from Stanford has demonstrated that they can also be applied to natural language tasks, in some instances producing quality outputs with less complexity and higher efficiency than architectures such as transformers. In today’s AI Atlas, I explore how Diffusion Models operate and explain where they create value today and how that might evolve moving forward.
🗺️ What are Diffusion Models?
A diffusion model is a type of AI that generates new data by starting with random noise and refining it step-by-step to create a clear and realistic output. For example, every time I use Midjourney to create header images for the AI Atlas, they begin as blurry shapes and gradually sharpen until becoming clear.
This process is learned by adding noise to real images and then teaching the model to reverse that process, removing the noise bit by bit to recreate the original image. These models are particularly good at generating high-quality images, producing detailed and diverse results. However, they are slow and require a lot of computational power because they have to go through many steps to create each image, making them less practical for real-time use.
The use of AI to create lifelike images is not unique to Diffusion Models. I previously explored GANs, which generate data through a competitive process between two models, which can lead to much faster results but are unstable and require extensive fine-tuning before becoming operational. This competition is used during training to improve results, and then GANs produce outputs in a single step. Diffusion Models, on the other hand, start with noise and slowly refine it in many steps to make clear and realistic outputs. This enables Diffusion Models to produce higher-quality images, but they are slower and require more computational power compared to GANs.
🤔 What is the significance of Diffusion Models and what are their limitations?
Diffusion Models have become extremely popular within Generative AI because they can produce highly detailed and diverse outputs with a reliably stable training process. Their versatility extends to various data types, including images, audio, and text, and they can be integrated with other architectures like transformers, which are great at capturing context and providing human-language interfaces, for enhanced performance. This combination of quality, stability, and adaptability positions Diffusion Models as a powerful and promising AI tool.
- High quality outputs: Diffusion Models are known for their ability to generate images and other outputs with unprecedented detail and realism. This high fidelity makes them suitable for applications where quality is more important than speed, such as when creating regular marketing content.
- Step-by-step refinement: Unlike some other GenAI systems such as transformers, which produce sentences sequentially, Diffusion Models go back and refine their outputs via an iterative process. This allows for finer control over generation, enabling stronger customization and allowing for adjustments based on specific needs.
- Flexibility in data types: As demonstrated by the aforementioned research, Diffusion Models are versatile in what they can generate. Beyond just images, they can be adapted to text, audio, and other forms of data, broadening their applicability across different industries.
However, the practical application of Diffusion Models is constrained by several key limitations, including:
- High resource cost: Training and processing Diffusion Models require significant computational power, often necessitating specialized hardware such as GPUs. This makes in-house Diffusion Models less accessible for enterprises without dedicated compute resources.
- Slow generation process: The step-by-step refinement process can be slow, especially compared to other generative models like GANs. This can be a drawback in applications where speed is critical, such as when building autonomous agents around core operations.
- Data intensity: While needing large datasets is common in AI, Diffusion Models are particularly data-hungry, requiring extensive high-quality datasets to achieve optimal performance. This can be challenging for applications where data is scarce or difficult to obtain, such as with protected healthcare data.
🛠️ Applications of Diffusion Models
Diffusion Models excel at producing high-quality data samples through their iterative de-noising process. This makes them particularly powerful for applications requiring high fidelity and fine-grained detail, such as:
- Content creation and product design: Diffusion Models can create realistic images from scratch, which are useful for creating custom visuals for marketing, advertising, and media production. For example, Common Sense Machines leverages Diffusion Models to develop high-quality textures for 3D models created using their Cube platform.
- Data augmentation: In machine learning, Diffusion Models are popular for creating synthetic data, or artificial inputs that mimic the distribution of real data. This is used to improve the performance of AI models when actual training data is hard to come across, such as when preserving consumer data privacy.
- Signal processing: Diffusion Models can be used to remove noise from signals generated by messy hardware in real-world situations. This is useful for cases such as improving the quality of voice recordings or when interpreting equipment readings to optimize factory processes.
Stay up-to-date on the latest AI news by subscribing to Rudina’s AI Atlas.