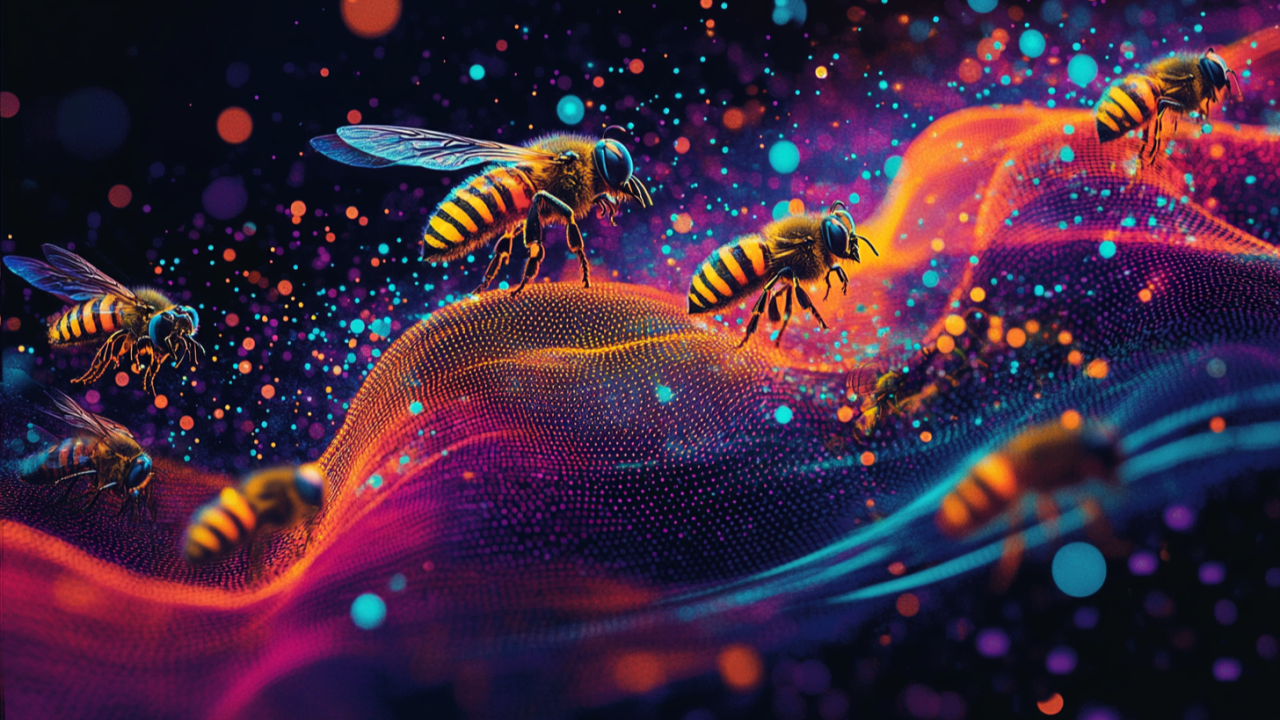
AI Atlas:
Collective Intelligence through Swarm Agents
Rudina Seseri
Last week, I spoke at MIT’s Imagination in Action Summit, where I had the opportunity to discuss the future trajectory of enterprise AI alongside titans of industry and academia. A central theme was the decentralization of AI, inspired by emerging open-source interoperability frameworks such as NANDA out of MIT’s Decentralized AI Program, headed by Ramesh Raskar. The NANDA framework builds on Anthropic’s Model Context Protocol (MCP) to create an “internet” of AI Agents, or intelligent systems designed to perceive their environment and make decisions autonomously, opening exciting new possibilities at the frontier of AI.
Longtime readers of this AI Atlas will recognize my enthusiasm for AI-native solutions that leverage multiple architectures or techniques in coordination, also known as ensemble methods, which exploit complementary strengths and result in higher accuracy compared to using a single model or AI agent. With frameworks such as NANDA and the MCP, we are witnessing the first phase of a global expansion in ensemble capabilities, as AI practitioners coordinate specialized models into large collectives — known as swarm agents — to achieve performance on enterprise use cases of unprecedented scale and complexity.
Thank you also to Cristian Jimenez Romero, Alper Yegenoglu, and Christian Blum, whose research on multi-agent systems I referenced while writing this Atlas!
🗺️ What are Swarm Agents?
A swarm agent is a collection of autonomous AI agents that operate in coordination toward a common goal, inspired by the behavior of insect swarms in nature (e.g., bees in a hive or ants building a colony). Each agent operates independently: gathering information, making decisions, and acting based on its specialized role. The swarm then collaborates as a whole, sharing insights and adapting to new situations to unlock capabilities impossible for a single AI agent to accomplish alone.
Unlike a traditional automation solution relying on rules-based centralized control, swarm agents are entirely decentralized. In other words, they do not need a single entity to tell them what to do. Instead, each individual agent continuously assesses its environment and adjusts its actions alongside its peers, much like a team that communicates in real-time without needing constant direction.
🤔 Why Swarm Agents Matter and Their Limitations
Swarm agents are a new paradigm in how businesses can approach automation, shifting from rigid predefined workflows to adaptive systems mirroring the way successful human teams or other natural systems operate. For enterprise leaders, this means better responsiveness and greater efficiency in managing complexity at scale. Further benefits of swarm agents include:
- Resilience: This structure makes it so each AI agent can focus on the task for which it is most optimized, reducing individual burdens. Additionally, even if a single agent fails or malfunctions, nearby agents in the swarm can step in and compensate for the missing capabilities.
- Scalability: As business operations expand, swarm agents can easily incorporate additional components. Each new agent joins the swarm and contributes to the shared intelligence, enabling seamless scaling across departments or geographies.
- Efficiency: Each agent in the swarm makes decisions locally, reducing delays and bottlenecks in complex operations. The system can quickly respond to fluctuating conditions, like abrupt market shifts, without awaiting new programming.
As the technology around swarm agents develops further, there are several key research areas for continued improvement:
- Complexity: Deploying and coordinating a swarm of agents is more complicated than traditional automation. It requires thoughtful design to anticipate how the system will behave in edge cases. Poorly designed swarms can produce erratic or unintended outcomes.
- Interpretability: Because swarm agents are autonomous, guardrails are needed to ensure that operators in high-impact use cases can understand the reasoning behind predictions and decisions made by the neural network.
- Data dependency: The effectiveness of swarms depends on access to accurate, timely data. Furthermore, because agents often exchange sensitive information, data must be closely protected to prevent breaches or misuse.
🛠️ Use cases of Swarm Agents
Swarm agents would be particularly powerful in environments where real-time responsiveness and adaptability are critical, such as:
- Financial operations: In banking and insurance, swarm agents could be used to monitor real-time transactions, flag potential compliance issues, and remediate with minimal human intervention. This would enable institutions to stay ahead of rapidly evolving regulations while reducing audit risk.
- Retail sales: E-commerce platforms could use swarms to tailor product recommendations and create personalized offers based on signals like a shopper’s browsing behavior, resulting in higher conversion rates and improved customer satisfaction.
- Supply chain/logistics: For example, if a disruption were to occur in a supply chain system, an agent swarm could automatically re-route deliveries or reallocate resources without waiting for centralized commands or manual intervention. This would improve delivery precision and reduce waste even in unpredictable conditions.
Stay up-to-date on the latest AI news by subscribing to Rudina’s AI Atlas.