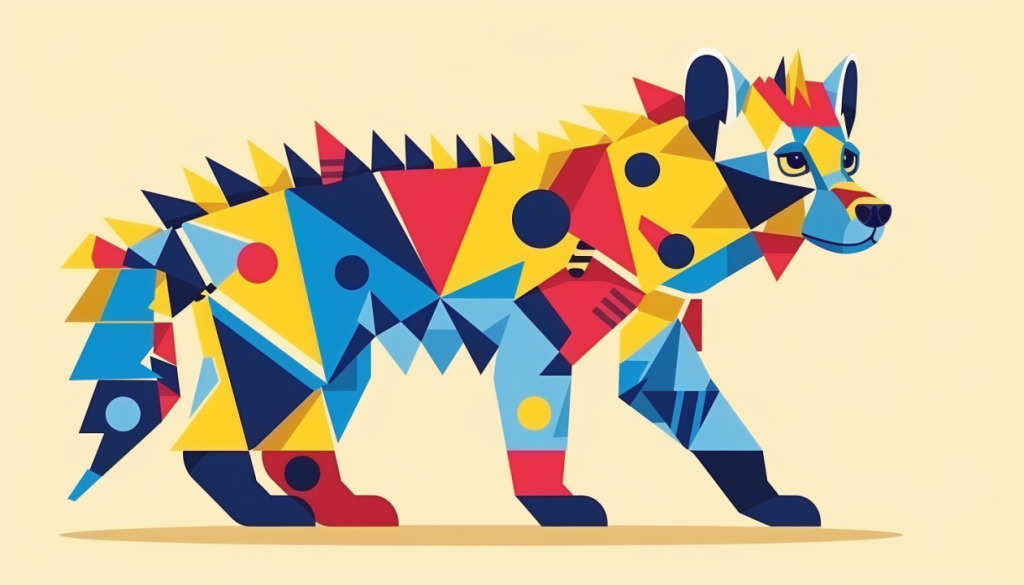
AI Atlas: Is Attention All You Need? A Look at Hyena
AI breakthroughs, concepts, and techniques that are tangibly valuable, specific, and actionable. Written by Glasswing Founder and Managing Partner, Rudina Seseri
Google Brain’s view that “attention is all you need” became the basis for generative AI, setting off the wave of technological innovation that surrounds us today. In the field of AI, “attention” refers to the ability of an AI model to compare inputs against each other, thus revealing the importance of individual data points. For example, in language models such as those that utilize transformers, from ChatGPT to Stable Diffusion, each word of a sentence is compared to every other word, allowing the model to develop a stronger grasp of the context behind an entire passage.
However, while transformer and attention models have undeniably driven a new wave in AI technologies, they have fundamental drawbacks that are difficult to solve. In particular, because attention requires comparing every data point to every other data point, the computational resources required increases massively with scale. Furthermore, the error rate of large language models, also known as their tendency to confidently output incorrect information, demonstrates diminishing positive returns relative to the amount of training data.
These problems beg the question: is attention the only way to unlock such incredible capabilities? Is it truly “all you need?” To this question enters a new challenger: Hyena.
🗺️ What is Hyena?
Hyena, developed by scientists at Stanford last year, is one potential alternative for the attention mechanism in large language models (LLMs). Rather than cross-referencing data pairs internally, Hyena de-emphasizes self-attention and instead works through a hierarchy of filters, called convolutional layers. In the context of convolutional neural networks, such layers extract features from the input data using filters that highlight specific characteristics. In Hyena, hyper-specific versions of these filters are leveraged to quickly process multiple aspects of a given input in parallel.
Employing filters rather than continuously readjusting model weights to match historical data means Hyena is less likely to experience overfitting, when an AI model learns the training data too well, capturing noise or random fluctuations instead of just the underlying pattern. This also enables Hyena to remember context over much longer data sequences, orders of magnitude larger than transformers today. Additionally, Hyena can utilize its convolutional layers to process such datasets at far greater speeds. At certain scales, for example, Hyena outperformed FlashAttention, the most speed-optimized attention model today, by a factor of 100x.
🤔 What is the significance of Hyena and what are its limitations?
The Hyena structure has demonstrated the ability to match the accuracy of attention at scale while reducing computational costs. Hyena’s ability to handle long sequences is extremely exciting. Imagine feeding ChatGPT an entire series of novels as context, and being able to ask specific questions about the data without the chatbot losing its place in the conversation. In the world of AI, where transformer- and attention-based architectures have dominated language models for years, Hyena represents a shift in thinking away from “more data makes better models,” instead looking towards alternative and more efficient solutions to the same problems.
- Resource-efficiency: As AI models increase in scale, they increase exponentially in compute costs. At one point, OpenAI was spending $700,000 per day on processing, a figure that will continue to rise. Hyena’s ability to save time and energy has the potential to unlock AI at new scale.
- Longer context: For the same amount of computational memory, Hyena can handle dramatically longer context. The original paper’s authors, for example, discuss the potential of loading in an entire textbook and then asking questions directly from the data.
- Simpler architecture: The evaluation of Hyena’s filters is fast and can be performed in parallel, making the architecture easier to work with and optimize to specific hardware.
However, at present, Hyena is nothing more than a model set in a limited testing environment. Much more research is needed in order to explore its full capabilities and limitations compared to much more mature models such as GPT-4 or Anthropic’s Claude.
- Results remain speculative: Early testing shows a 20% improvement on resource costs relative to some attention-based systems, but more testing is necessary to understand what this looks like on a wider variety of datasets and use cases.
- Error rate and hallucinations: The failings of transformers such as the GPT family regarding hallucinations, or the tendency to confidently output false information, is very well-documented. Hyena, being still in early development, will need to be benchmarked against the same level of testing.
- Wider structure and interoperability: As Hyena develops, it will also be necessary to understand if it functions as a “plug and play” solution over attention-based architectures or whether an entirely new ecosystem would need to be built up to fully leverage its strengths within business applications.
🛠️ Applications of Hyena
Hyena is an intriguing, albeit still speculative, proposal for an efficient alternative to traditional attention architectures used across AI tasks. Its technical promise highlights the potential for further research into more efficient deep learning models to open up a new wave of tools across use cases such as:
- Natural Language Processing (NLP): Human language is a complex target for AI models, which is why LLMs require so much power and data to operate. Improving performance at large scales could lead to more powerful chatbots and systems such as ChatGPT.
- Computer Vision: Longer context lengths could translate to the ability to analyze more detailed images, or improved accuracy in object recognition. For example, a security system fed an entire day’s worth of footage may be able to pick up critical patterns that a smaller system would miss.
- Time series data: Sequential, time-series data is common in a wide variety of industries, from machine monitoring to healthcare and even the stock market. Increasing not just the amount of data capable of being analyzed but also the length of time during which to collect data would improve model accuracy. For example, a hospital could use a patient’s lifetime health record as a source of truth for diagnoses.
Stay up-to-date on the latest AI news by subscribing to Rudina’s AI Atlas.