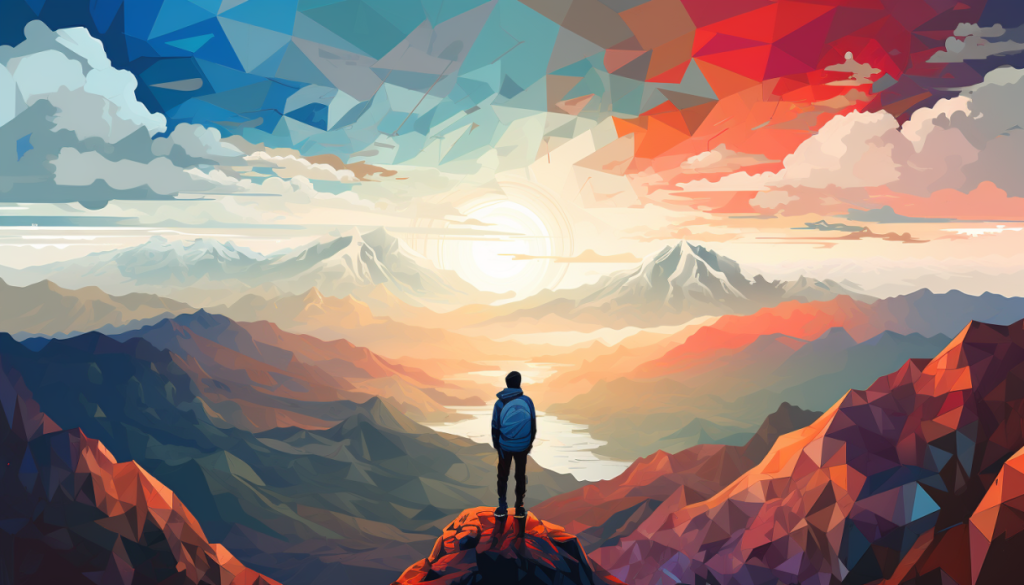
What Your Children Can Do That Generative AI Still Cannot
Rudina Seseri
Last month, researchers at UC Berkeley published a study on Large Language Models (LLMs) such as ChatGPT, comparing these systems’ learning abilities with those of human children. Discussions around artificial intelligence primarily revolve around the concept of AI Agents, which I previously defined as software systems designed to perceive their environment, reason about it, make decisions, and take actions to achieve specific objectives autonomously. The study expands on this definition by suggesting we should think of LLMs not as replacements for human intelligence, but as highly complementary tools. Below, I dive deeper into the research and explore the implications of this perspective.
🗺️ Overview of the research
In terms of learning, the researchers compare LLMs to human children, particularly in their ability to learn from and replicate observed behavior. However, while LLMs can mimic certain behaviors or patterns, it is widely accepted that they do not have the same depth of understanding or the ability to innovate as humans do. At a fundamental level, these systems are merely advanced mechanisms designed to build sentences one word at a time. They are excellent at aggregating and pattern-matching large datasets, but they lack the capacity for true innovation or causal understanding beyond their training data.
The paper thus suggests that while LLMs can help in understanding which cognitive capacities can be acquired through cultural transmission, they are not replacements for human creativity or problem-solving abilities. Rather, the models are better seen as tools for cultural transmission and production, akin to the printing press or the Internet.
Furthermore, LLMs struggle with tasks that demand causal reasoning or the generation of novel ideas beyond their training data, something that comes easily to children (as anyone who has seen their child play pretend can attest to). Compared with systems designed for perception and action – such as reinforcement learning architectures in robotics, which alter their models based on contact with the outside world and thus generate novel actions – typical large language and vision models rely on relatively simple statistical inference applied to large amounts of pre-existing data. This means that LLMs act reactively, based on what has happened in the past, rather than dynamically adjusting to new data and making long-term decisions.
🤔 What is the significance of these findings, and what does GenAI mean for us today?
The enterprise value of AI and its economic impact will continue to grow in scale, but not in the way that many expect. For a comparison: in the middle ages, alchemists spent their lives trying to turn lead into gold. Today these pursuits have revealed the principles of chemistry, a field that has completely transformed human society in every aspect of life; however, the creation of gold from lead is still something we cannot easily do. Likewise, there are many things that Generative AI can do extremely well today, but those who view the technology as a panacea for every societal ill — figuratively, believing that AI can turn lead into gold — will simply find themselves disappointed.
So, what can Generative AI do well? Per learnings from the original research, it is helpful to not think of an LLM as comparable to coworkers on your team; rather, it is a powerful drafting and summarization tool with a long tail of resulting innovations. Building a successful enterprise app utilizing Generative AI requires a deep understanding of a vertical, what errors matter, and the extent to which those errors matter.
🛠️ Applying these learnings practically
Understanding the differences between Large Language Models and humans can provide insights into both natural intelligence and artificial intelligence and help in designing more advanced AI systems. For example, consider the following use cases:
Risk Management and Decision Support: By processing and synthesizing large amounts of data, LLMs can provide insights for risk analysis and strategic decision-making, helping leaders make informed choices.
Automated Programming and Debugging: LLM co-pilots substantially eliminate repetitive work related to coding tasks, but it is still up to programmers to understand and define problems worth solving. Mindfully leveraging AI systems can help write, review, and debug code, thus speeding up the development process, reducing the likelihood of errors, and lowering the technical barrier to software development.
Process Automation: Routine administrative tasks such as scheduling, email management, and document processing can be automated using LLMs, leading to increased efficiency and reduced administrative overhead. At the same time, human operators are empowered to dedicate more time towards strategy and long-term planning.
We are fortunate to be witnessing the meteoric rise of artificial intelligence in real-time, as it becomes a wholly ubiquitous technology in global affairs. I have been excited by the possibilities of AI for over a decade, and LLMs are proving to take this revolution even further.