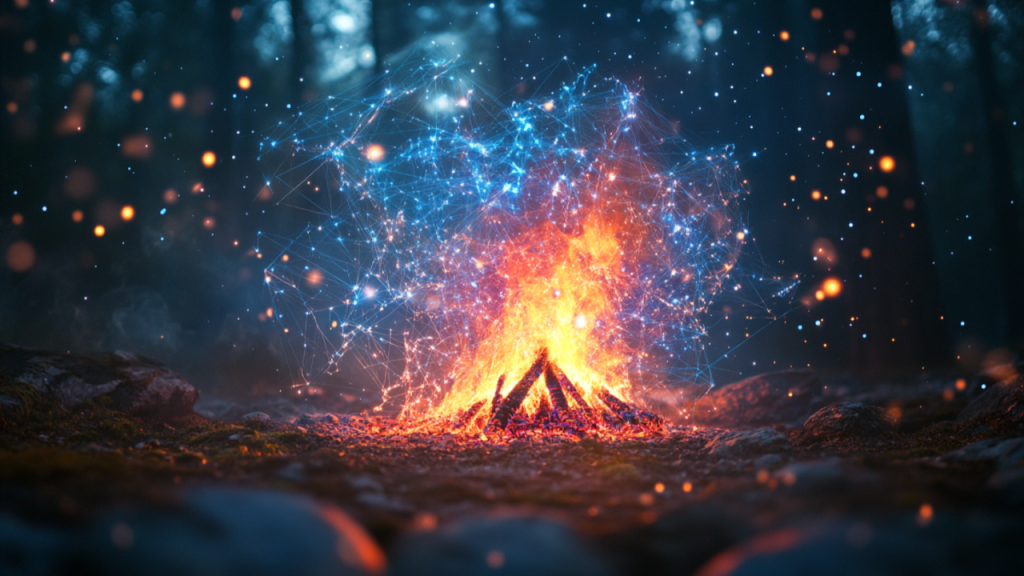
AI Atlas:
How Can We Make AI More Truthful?
Rudina Seseri
Large Language Models (LLMs) like ChatGPT and Claude are trained to generate human-like text and follow natural language instructions. However, a famously persistent problem with these models is their tendency to generate false or misleading information – a phenomenon referred to as “AI hallucination.” For businesses, relying on incorrect AI-generated outputs can lead to costly mistakes and painful reputational damage. Unfortunately, aligning AI with the “truth” is often a difficult task, requiring intricate coordination between algorithms, the end users, and the data used for training in the first place.
In research published last month, Meta‘s AI team announced a novel algorithm designed to address the problem of AI hallucinations. Known as FLAME, the technique aims to incorporate authenticity into the core of an LLM prior to deployment. In today’s AI Atlas, I dive further into this research and explore its potential value for business use cases.
🗺️ What is FLAME?
FLAME, a creative abbreviation for “Factuality-Aware Alignment for Large Language Models,” is a specialized algorithm designed to reduce hallucinations in AI systems. The technique focuses on priming an AI model to produce more accurate and reliable outputs while maintaining its ability to follow complex instructions. In doing so, FLAME ensures that an LLM can deliver trustworthy responses across a range of business applications, from customer service to high-stakes industries such as finance and law.
Traditional approaches to AI alignment generally revolve around two main stages: Supervised Fine-Tuning, where pre-existing knowledge is integrated into the model to improve its performance in specific contexts, and Reinforcement Learning, where a model undergoes trial and error to optimize a desired behavior. However, these techniques have inherent biases which ultimately and inadvertently encourage the generation of false claims. To address this limitation, FLAME incorporates a simplified process known as Direct Preference Optimization, which leverages human feedback to bring fact-based accuracy to the core of a model. This enables FLAME-enhanced models to generate significantly higher-quality outputs without requiring additional processing.
🤔 What is the significance of FLAME and what are their limitations?
FLAME addresses one of the most pressing challenges that business face when adopting AI: trust. Meta’s researchers demonstrated that, after applying FLAME to an LLM, the model both followed instructions better and produced materially more factual responses. In other words, by restricting the spread of inaccurate data during model training, FLAME shows enormous potential for enterprises seeking to use AI with greater confidence.
- Enhanced factual accuracy: FLAME reduces the frequency of hallucinations, ensuring that an LLM generates reliable and trustworthy outputs. This is valuable for all industries, especially as AI approaches use cases at the business core.
- Maintaining performance: FLAME ensures that AI remains accurate without sacrificing the model’s speed or ability to follow instructions.
- Adaptability: FLAME’s methodology can be applied to diverse tasks across industries, making it a versatile solution for businesses developing AI in new operational contexts.
However, it is important to recognize that FLAME is not a perfect solution. AI alignment is a complex process, and while FLAME significantly reduces hallucinations it does not eliminate them entirely. The researchers behind the model acknowledged a few areas for continued study:
- Residual hallucinations: FLAME reduces errors significantly over a control model, but is not yet 100% accurate. Businesses must still validate AI-generated outputs in critical contexts.
- Fine-tuning on specific tasks: Given that some tasks, particularly in regulated industries, have lower fault tolerance and higher thresholds for acceptable performance, the use of FLAME may require fine-tuning for use cases close to the business core.
- Complexity implementation: Developing AI alignment is an involved process, and deploying FLAME may be too resource-intensive for smaller organizations.
🛠️ Applications of FLAME
FLAME is a significant step forward in making AI a more reliable and effective tool for businesses where the threshold for acceptable performance is high, particularly in use cases such as:
- Generating data-driven insights: Business analysts could rely on FLAME-enhanced models to summarize critical reports, draw insights, or draft strategic recommendations.
- Sales automation: Businesses can deploy AI sales agents with FLAME to provide accurate answers to customer queries, reducing the risk of misinformation.
- AI agents in regulated industries: For healthcare and legal use cases, where hallucinations and errors have significant regulatory consequences, FLAME could ensure that AI outputs align with verified knowledge.
Understanding the acceptable error rate for an AI application is just one of several key considerations. Interested in learning more about the path that the industry’s most successful enterprises are taking to adopt AI? Glasswing Ventures recently published our proprietary AI Value Creation Framework to visualize the key considerations that businesses should be aware of.
Stay up-to-date on the latest AI news by subscribing to Rudina’s AI Atlas.