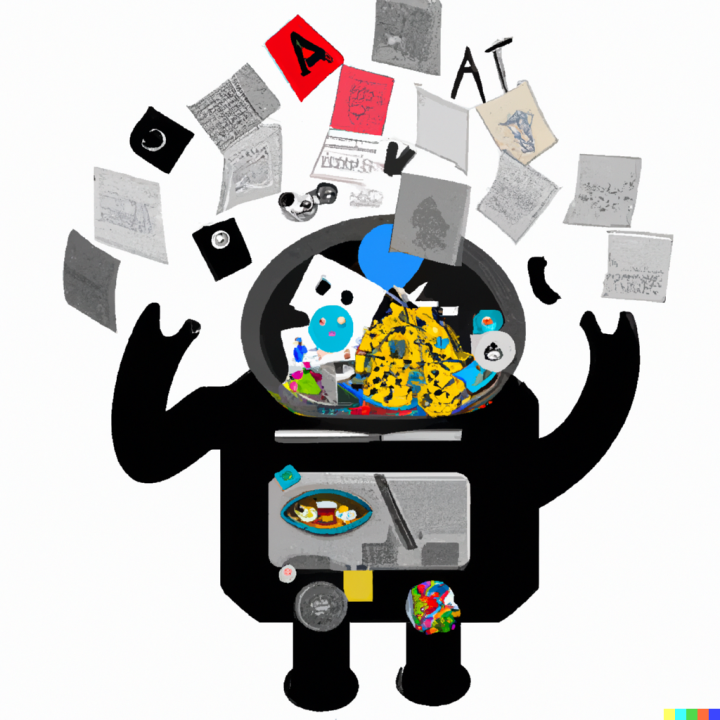
AI Atlas #23:
OpenAI’s CLIP
Rudina Seseri
🗺️ What is OpenAI’s CLIP?
OpenAI’s CLIP (Contrastive Language-Image Pre-training) is a neural network that is trained to understand images paired with natural language. It represents a significant advancement in both vision and language understanding by merging the capabilities of both in a single model. CLIP is a multimodal model, meaning it is designed to process and understand information from multiple types of data/modalities simultaneously, such as text, images, audio, video, etc.
Unlike traditional models that are fine-tuned for specific tasks, CLIP learns from vast amounts of internet images and their accompanying textual descriptions. By maximizing the correlation between an image’s features and its description, it bridges the gap between vision and language in a unified model. This methodology enables CLIP to perform “zero-shot learning.”
To understand how CLIP works, we can imagine a huge photo album of pictures with corresponding short notes about each. If you then studied each picture and corresponding caption for a long enough time, you would eventually learn to perfectly match a photo to a caption. If you were then asked “which note from the album best describes this picture?”, even if they’ve never seen this photo before, you can make a really good guess based on the features of the photos you previously studied. That’s because you have become really good at understanding both pictures and words and seeing how they relate. Put simply, CLIP is a computer program that is great at matching images with words, through a similar learning process.
🤔 Why CLIP Matters and Its Shortcomings
OpenAI’s CLIP has numerous significant implications across broader AI including:
Bridging Vision and Language: Traditionally, computer programs and AI models were either good at understanding images or text, but not both. CLIP is a leap forward because it is trained to understand and link both images and words. This opens up new possibilities for tasks that require a blend of visual and textual understanding.
Versatility: Unlike many AI models that are trained for one specific task, CLIP can tackle a wide range of tasks without needing to be retrained. This is achieved through zero-shot learning, as I covered in a previous AI Atlas. For instance, it can categorize images, generate descriptions, or even answer questions about visuals – all without specialized training for each function.
Reduced Need for Large Labeled Datasets: Training traditional AI models, especially for visual tasks, often requires massive amounts of carefully labeled data. CLIP’s approach reduces the need for this, as it can understand and generalize from the vast and varied data for which it was trained.
Potential for New Applications: With its ability to associate text and images, CLIP can be used in innovative ways, like better search engines for images, assisting visually impaired individuals, aiding in educational tools, and more.
As with all breakthrough models, there are limitations to OpenAI CLIP including:
Over-reliance on Textual Prompts: The performance of CLIP can vary based on the phrasing of textual prompts. Slight variations in how a question or instruction is worded can lead to different results, which means users may need to fine-tune their prompts to get the desired output. This is commonly referred to as “prompt engineering”.
Generalist vs. Specialist: Although CLIP is versatile and can handle a wide range of tasks, it might not always outperform models that are specifically trained (fine-tuned) for a particular task.
Potential for Biases: Like many large-scale AI models, CLIP is trained on vast amounts of internet data. This means it might inherit and perpetuate biases present in its training data, leading to biased or unfair outputs in certain scenarios.
Mismatched Associations: While CLIP is trained to associate images with textual descriptions, it can sometimes make associations that humans might find non-intuitive or incorrect. This is due to the differences in how models perceive patterns compared to human judgment.
Lack of Reasoning: While CLIP can associate text and images, it does not “understand” in the human sense. It cannot provide deep reasoning or explanations for its outputs, which can be crucial in some applications.
đź› Use Cases of OpenAI CLIP
Through its ability to bridge vision and language, OpenAI has numerous applications across domains including:
Image Classification: Given a textual description, CLIP can categorize or label images, making it valuable for tasks like photo organization, content moderation, or product categorization.
Content Moderation: Platforms can use CLIP to identify and filter out inappropriate content by associating images with specific textual descriptions related to unwanted content.
Visual Search Engines: Users can search for images using natural language queries, and CLIP can retrieve relevant images based on the textual descriptions.
E-commerce: Online retailers can use CLIP to improve product search, allowing users to search for products using descriptive text and retrieving visually matching items.
Augmented Reality: AR applications are enabled by providing real-time textual information about visual objects in the user’s environment.
Medical Imaging: While specialized models are typically preferred, in scenarios with limited labeled data, CLIP can assist in identifying or categorizing medical images based on textual descriptions.
Looking forward, OpenAI’s CLIP looks promising as it paves the way for more integrated AI applications that bridge vision and language. Researchers are likely to improve upon its architecture, making it more accurate and efficient. As the technology matures, we can expect its adoption in diverse sectors, from e-commerce to healthcare, enhancing user experience and decision-making processes. Moreover, CLIP’s approach may inspire new AI models that generalize across multiple data types, signaling a shift from task-specific models to more versatile AI solutions.