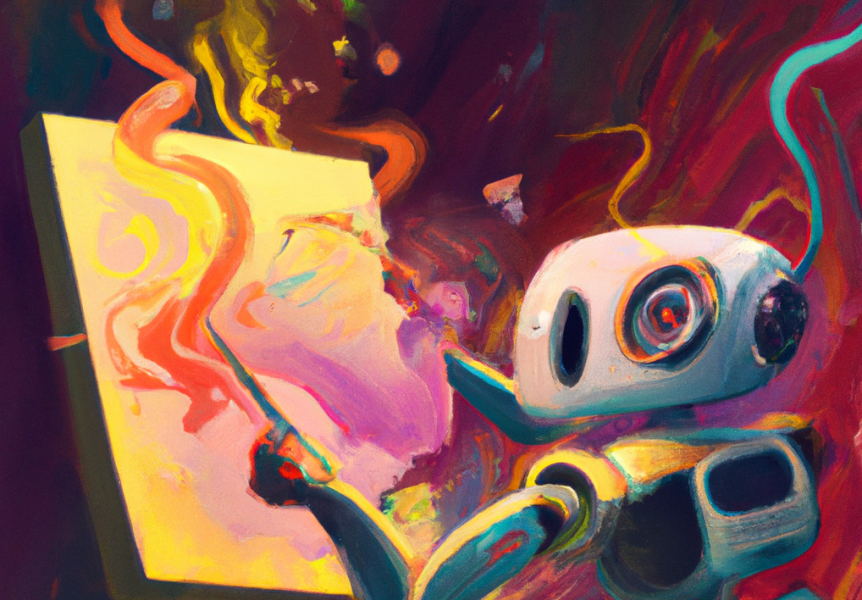
Multimodal AI:
Turning paintings into songs, and what that means for your business
Rudina Seseri
Earlier this month, researchers at the National University of Singapore unveiled NExT-GPT, an open-source Large Language Model (LLM) capable of accepting and delivering content across various forms, called “modalities”, including text, images, videos, and audio. This week, OpenAI announced that ChatGPT will be able to accept audio and visual inputs from users, and even reply in a synthetic voice. While traditional LLMs accept and produce only text-based responses, these innovations allow for greater flexibility in terms of data modality, a concept referred to as multimodality.
🗺️ What is multimodal AI?
NExT-GPT and OpenAI’s new features for ChatGPT are both examples of composite AI models, made up of several different AI “building blocks” around a core LLM. This can be imagined as a company with divisions specializing in audio, images, videos, and text, all of whom report to the CEO. The divisions consume relevant inputs and summarize them in a way that the central model understands, and its response is then passed to the relevant departments for output.
This structure unlocks the ability to work across formats; for example, you can create a song to fit promotional artwork or send a text message to request an illustrative video. The model will first process your input through the correct channel, create a response using the central LLM, and then transform the response into the desired form of output.
🤔 Why multimodality matters and its shortcomings
As I covered in August in the context of OpenAI CLIP, the opportunity in multimodal artificial intelligence is to make AI more versatile and human-like in its capabilities including:
Uniting vision, language, and audio: The ability to accept inputs and deliver outputs in any modality is a significant advancement that allows for seamless communication and content generation across various media types, making it valuable in industries where diverse modalities are essential, such as marketing, where customers are engaged with visuals across social media but also text content on newsletters and websites.
Efficient model tuning: The modular structure of multimodal AI, where different departments handle different media, allows for flexibility over time, as you don’t need to re-train the entire system with new integrations. This efficiency is also valuable because it allows the overall model to build upon its existing knowledge, potentially lowering the entry barrier for businesses looking to leverage multimodal AI.
Enhanced user experiences: “Any-to-Any” multimodal capabilities can significantly improve user experiences across industries. For example, in e-commerce, users can quickly express their preferences using images or voice, making the shopping process more efficient and enjoyable.
However, while OpenAI’s newest features represent a significant advancement in how we look at multimodal AI capabilities, its current state brings many key limitations:
Technological Nascency: ChatGPT has yet to fully integrate image and video generation and parent company OpenAI sits on many standing questions relating to safety and privacy. NExT-GPT, an open-source alternative, needs far more research to be production-ready with performance comparable to the now-outdated GPT-2.
Scalability: Each new modality introduces complexities in data pre-processing, fine-tuning, and training. Imagine deploying ChatGPT to handle text for an e-commerce platform. As the company grows, you decide to incorporate image and video search as well, but this amplifies the complexity as each product now has associated images, text, and video. Additionally, these models require large amounts of compute, which adds up to a substantial cost tradeoff in exchange for accuracy.
Lack of explainability: Like all deep neural networks, ChatGPT and NExT-GPT run into challenges relating to explainability and transparency within its inner layers. Thus, it is difficult to identify biases or errors behind the models’ assumptions and predictions.
🛠️ Applications of multimodal AI
The ability to understand and generate content in multiple modalities opens up a wide range of business use-cases across various industries. Here are some examples:
Content Creation and Marketing: Using a multimodal AI model, users can generate blog posts, articles, social media posts, and marketing materials across formats. This enables the creation of personalized content, tailored to individual users based on their preferences and behaviors.
Advanced Data Analysis: Businesses in market research can harness multimodal capabilities on diverse sources of data to gain deeper insights and make better decisions.
Security: Using NExT-GPT, security threats can be identified by analyzing multimodal data, including surveillance footage and audio recordings.
Computer-aided design (CAD): Leveraging the creative ability of LLMs alongside image recognition can allow for rapid prototyping cycles and greater efficiency in the manufacturing sector.
Ultimately, this month’s innovations represent a cognitive shift in how we view the long-term potential of Large Language Models. As research and development continue across the industry, we will see more technologies appear that solve existing LLM limitations and establish a framework capable of handling production use-cases. Businesses that embrace these technologies will be able to enhance customer experiences, streamline operations, and unlock new revenue streams.